Default model - make time-series by assimilating all test months into trained model¶
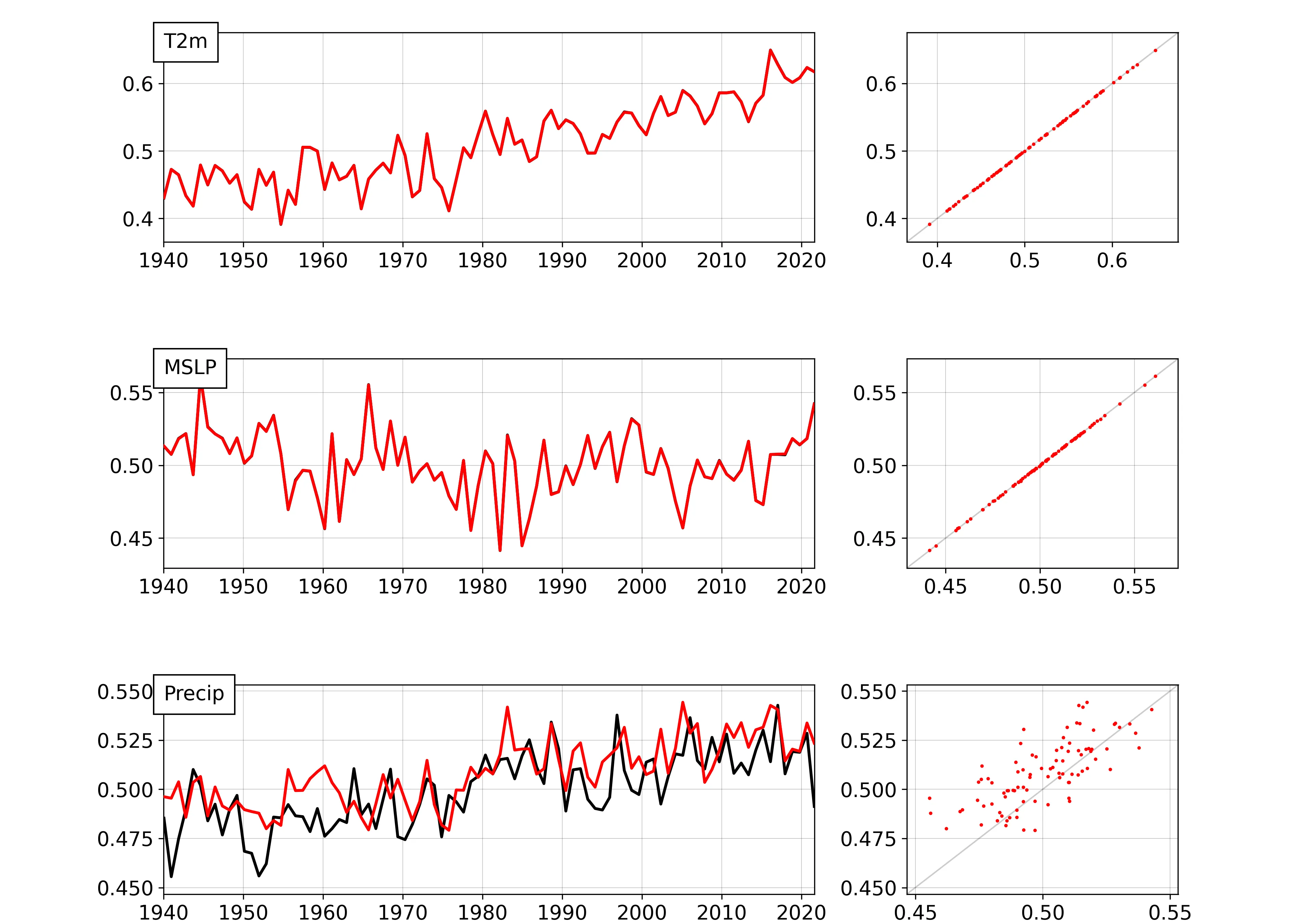
Global mean series (black original, red DCVAE output) and scatterplots for each output variable. T2m and MSLP were assimilated, Precipitation wasn’t - it’s model output.¶
Script assimilate_multi.py make the time-series validation figure - global means of each variable and scatterplots - when assimilating fields from the test dataset.
By default, it will use the test set, but the –training argument will take months from the training set instead of the test set. By default, it won’t assimilate anything, specify variables to assimilate as arguments (so the figure above has arguments --T2m
and --MSLP
). The –epoch argument specifies the model epoch to use.
#!/usr/bin/env python
# Plot fit statistics for all the test cases
import os
import sys
import numpy as np
# Supress TensorFlow moaning about cuda - we don't need a GPU for this
# Also the warning message confuses people.
os.environ["TF_CPP_MIN_LOG_LEVEL"] = "3"
import tensorflow as tf
import tensorflow_probability as tfp
import datetime
from statistics import mean
from specify import specification
from ML_models.default.makeDataset import getDataset
from ML_models.default.autoencoderModel import getModel
from ML_models.default.gmUtils import (
computeScalarStats,
plotScalarStats,
)
import argparse
parser = argparse.ArgumentParser()
parser.add_argument("--epoch", help="Epoch", type=int, required=False, default=500)
parser.add_argument(
"--startyear", help="First year to plot", type=int, required=False, default=None
)
parser.add_argument(
"--endyear", help="Last year to plot", type=int, required=False, default=None
)
parser.add_argument(
"--xpoint",
help="Extract data at this x point",
type=int,
required=False,
default=None,
)
parser.add_argument(
"--ypoint",
help="Extract data at this y point",
type=int,
required=False,
default=None,
)
parser.add_argument(
"--training",
help="Use training months (not test months)",
dest="training",
default=False,
action="store_true",
)
for field in specification["outputNames"]:
parser.add_argument(
"--%s" % field,
help="Fit to %s?" % field,
default=False,
action="store_true",
)
for field in specification["outputNames"]:
parser.add_argument(
"--%s_mask" % field,
help="Mask for fit to %s?" % field,
type=str,
default=None,
)
parser.add_argument(
"--iter",
help="No. of iterations",
type=int,
required=False,
default=100,
)
args = parser.parse_args()
args_dict = vars(args)
# Load the masks, if specified
fitted = {}
masked = {}
for field in specification["outputNames"]:
masked[field] = None
if args_dict["%s_mask" % field] is not None:
masked[field] = np.load(args_dict["%s_mask" % field])
fitted[field] = args_dict[field]
# Set up the test data
purpose = "Test"
if args.training:
purpose = "Train"
testData = getDataset(specification, purpose=purpose)
testData = testData.batch(1)
# Load the trained model
autoencoder = getModel(specification, optimizer=None, epoch=args.epoch)
def decodeFit():
result = 0.0
generated = autoencoder.generate(latent, training=False)
for field in specification["outputNames"]:
if fitted[field]:
field_idx = specification["outputNames"].index(field)
mask = masked[field]
if mask is not None:
mask = tf.constant(mask, dtype=tf.float32)
result = result + tf.reduce_mean(
tf.keras.metrics.mean_squared_error(
tf.boolean_mask(generated[0, :, :, field_idx], mask),
tf.boolean_mask(target[:, :, field_idx], mask),
)
)
else:
result = result + tf.reduce_mean(
tf.keras.metrics.mean_squared_error(
generated[:, :, :, field_idx], target[:, :, field_idx]
)
)
return result
# Go through the data and get the scalar stat for each test month
all_stats = {}
all_stats["dtp"] = []
all_stats["target"] = {}
all_stats["generated"] = {}
for case in testData:
if specification["outputTensors"] is not None:
target = tf.constant(case[2][0], dtype=tf.float32)
else:
target = tf.constant(case[1][0], dtype=tf.float32)
latent = tf.Variable(autoencoder.makeLatent())
if any(fitted.values()):
loss = tfp.math.minimize(
decodeFit,
trainable_variables=[latent],
num_steps=args.iter,
optimizer=tf.optimizers.legacy.Adam(learning_rate=0.1),
)
generated = autoencoder.generate(latent, training=False)
stats = computeScalarStats(
specification,
case,
generated,
)
all_stats["dtp"].append(stats["dtp"])
for key in stats["target"].keys():
if key in all_stats["target"]:
all_stats["target"][key].append(stats["target"][key])
all_stats["generated"][key].append(stats["generated"][key])
else:
all_stats["target"][key] = [stats["target"][key]]
all_stats["generated"][key] = [stats["generated"][key]]
# Make the plot
plotScalarStats(all_stats, specification, fileName="assimilate_multi.webp")
Utility functions used in the plot
# Model utility functions
import os
import sys
import numpy as np
import datetime
import iris
import tensorflow as tf
from tensorflow.core.util import event_pb2
from tensorflow.python.framework import tensor_util
import matplotlib
from matplotlib.backends.backend_agg import FigureCanvasAgg as FigureCanvas
from matplotlib.figure import Figure
from matplotlib.patches import Rectangle
from matplotlib.lines import Line2D
import cmocean
from utilities import plots, grids
# Convenience function to make everything a list
# lists stay lists, scalars become len=1 lists.
def listify(input):
if isinstance(input, str):
input = [input]
else:
try:
iter(input)
except TypeError:
input = [input]
else:
input = list(input)
return input
# Load the history of a model from the Tensorboard logs
def loadHistory(LSC, offset=-1, max_epoch=None):
history = {}
summary_dir = "%s/DCVAE-Climate/%s/logs/Training" % (os.getenv("SCRATCH"), LSC)
Rfiles = os.listdir(summary_dir)
Rfiles.sort(key=lambda x: os.path.getmtime(os.path.join(summary_dir, x)))
filename = Rfiles[offset]
path = os.path.join(summary_dir, filename)
serialized_records = tf.data.TFRecordDataset(path)
history["epoch"] = []
for srecord in serialized_records:
event = event_pb2.Event.FromString(srecord.numpy())
for value in event.summary.value:
t = tensor_util.MakeNdarray(value.tensor)
if not value.tag in history.keys():
history[value.tag] = []
if value.tag == "OutputNames":
history[value.tag] = t
continue
if len(history[value.tag]) < event.step + 1:
history[value.tag].extend(
[None] * (event.step + 1 - len(history[value.tag]))
)
history[value.tag][event.step] = t
if len(history["epoch"]) < event.step + 1:
history["epoch"].extend([None] * (event.step + 1 - len(history["epoch"])))
history["epoch"][event.step] = event.step
ymax = 0
ymin = 1000000
hts = {}
n_epochs = len(history["Train_loss"])
if max_epoch is not None:
n_epochs = min(max_epoch, n_epochs)
hts["epoch"] = list(range(n_epochs))[1:]
hts["epoch"] = history["epoch"]
for key in history:
if key == "OutputNames":
hts[key] = [str(t, "utf-8") for t in history[key]]
else:
hts[key] = [abs(t) for t in history[key][1:n_epochs] if t is not None]
for key in ("Train_logpz", "Train_logqz_x", "Test_logpz", "Test_logqz_x"):
ymax = max(ymax, max(hts[key]))
ymin = min(ymin, min(hts[key]))
return (hts, ymax, ymin, n_epochs)
# Choose colourmap based on variable name
def get_cmap(name):
if name == "PRATE" or name == "Precip":
return cmocean.cm.tarn
elif name == "MSLP":
return cmocean.cm.diff
else:
return cmocean.cm.balance
# Plot a single-field validation figure for the autoencoder.
def plotValidationField(specification, input, output, year, month, fileName):
nFields = specification["nOutputChannels"]
# Make the plot
figScale = 3.0
wRatios = (2, 2, 1.25)
if specification["trainingMask"] is not None:
wRatios = (2, 2, 1.25, 1.25)
fig = Figure(
figsize=(figScale * sum(wRatios), figScale * nFields),
dpi=100,
facecolor=(1, 1, 1, 1),
edgecolor=None,
linewidth=0.0,
frameon=True,
subplotpars=None,
tight_layout=None,
)
canvas = FigureCanvas(fig)
font = {
"family": "DejaVu Sans",
"sans-serif": "DejaVu Sans",
"weight": "normal",
"size": 12,
}
matplotlib.rc("font", **font)
# Each variable a row in it's own subfigure
subfigs = fig.subfigures(nFields, 1, wspace=0.01)
if nFields == 1:
subfigs = [subfigs]
for varI in range(nFields):
ax_var = subfigs[varI].subplots(
nrows=1, ncols=len(wRatios), width_ratios=wRatios
)
# Left - map of target
varx = grids.E5sCube.copy()
varx.data = np.squeeze(input[-1][:, :, :, varI].numpy())
varx.data = np.ma.masked_where(varx.data == 0.0, varx.data, copy=False)
if varI == 0:
ax_var[0].set_title("%04d-%02d" % (year, month))
ax_var[0].set_axis_off()
x_img = plots.plotFieldAxes(
ax_var[0],
varx,
vMax=1.25,
vMin=-0.25,
cMap=get_cmap(specification["outputNames"][varI]),
)
# Centre - map of model output
vary = grids.E5sCube.copy()
vary.data = np.squeeze(output[:, :, :, varI].numpy())
vary.data = np.ma.masked_where(varx.data == 0.0, vary.data, copy=False)
ax_var[1].set_axis_off()
ax_var[1].set_title(specification["outputNames"][varI])
x_img = plots.plotFieldAxes(
ax_var[1],
vary,
vMax=1.25,
vMin=-0.25,
cMap=get_cmap(specification["outputNames"][varI]),
)
# Third - scatter plot of input::output - where used for training
ax_var[2].set_xticks([0, 0.25, 0.5, 0.75, 1])
ax_var[2].set_yticks([0, 0.25, 0.5, 0.75, 1])
if specification["trainingMask"] is not None:
varxm = varx.copy()
varym = vary.copy()
mflat = specification["trainingMask"].numpy().squeeze()
varxm.data = np.ma.masked_where(mflat == 1, varxm.data, copy=True)
varym.data = np.ma.masked_where(mflat == 1, varym.data, copy=True)
plots.plotScatterAxes(
ax_var[2], varxm, varym, vMin=-0.25, vMax=1.25, bins="log"
)
else:
plots.plotScatterAxes(
ax_var[2], varx, vary, vMin=-0.25, vMax=1.25, bins="log"
)
# Fourth only if masked - scatter plot of input::output - where masked out of training
if specification["trainingMask"] is not None:
ax_var[3].set_xticks([0, 0.25, 0.5, 0.75, 1])
ax_var[3].set_yticks([0, 0.25, 0.5, 0.75, 1])
mflat = specification["trainingMask"].numpy().squeeze()
varx.data = np.ma.masked_where(mflat == 0, varx.data, copy=True)
vary.data = np.ma.masked_where(mflat == 0, vary.data, copy=True)
plots.plotScatterAxes(
ax_var[3], varx, vary, vMin=-0.25, vMax=1.25, bins="log"
)
fig.savefig(fileName)
def plotTrainingMetrics(
specification, hts, fileName="training.webp", chts=None, aymax=None, epoch=None
):
fig = Figure(
figsize=(15, 5),
dpi=100,
facecolor=(1, 1, 1, 1),
edgecolor=None,
linewidth=0.0,
frameon=True,
subplotpars=None,
tight_layout=None,
)
canvas = FigureCanvas(fig)
font = {
"family": "DejaVu Sans",
"sans-serif": "DejaVu Sans",
"weight": "normal",
"size": 12,
}
matplotlib.rc("font", **font)
def addLine(ax, dta, key, col, z, idx=0, rscale=1):
dtp = [listify(x)[idx] for x in dta[key] if len(listify(x)) > idx]
dta2 = [
dta["epoch"][i]
for i in range(len(dta[key]))
if len(listify(dta[key][i])) > idx
]
ax.add_line(
Line2D(
dta2,
np.array(dtp) * rscale,
linewidth=2,
color=col,
alpha=1.0,
zorder=z,
)
)
# Three subfigures
# Left - for the overall loss
# Centre, for the RMS components
# Right, for the KL-divergence components
subfigs = fig.subfigures(1, 3, wspace=0.07)
# Left - Main loss
ymaxL = max(1, max(hts["Train_loss"] + hts["Test_loss"]))
if chts is not None:
ymaxL = max(ymaxL, max(chts["Train_loss"] + chts["Test_loss"]))
if aymax is not None:
ymaxL = aymax
subfigs[0].subplots_adjust(left=0.2)
ax_loss = subfigs[0].subplots(nrows=1, ncols=1)
ax_loss.set_xlim(left=-1, right=epoch + 1, auto=False)
ax_loss.set_ylim(bottom=0, top=ymaxL, auto=False)
ax_loss.set_ylabel("Overall loss")
ax_loss.set_xlabel("epoch")
ax_loss.grid(color=(0, 0, 0, 1), linestyle="-", linewidth=0.1)
addLine(ax_loss, hts, "Train_loss", (1, 0.5, 0.5, 1), 10)
addLine(ax_loss, hts, "Test_loss", (1, 0, 0, 1), 20)
if chts is not None:
addLine(ax_loss, chts, "Train_loss", (0.5, 0.5, 1, 1), 10)
addLine(ax_loss, chts, "Test_loss", (0, 0, 1, 1), 20)
# Centre, plot each RMS component as a separate subplot.
comp_font_size = 10
nvar = len(hts["OutputNames"])
# Layout n plots in an (a,b) grid
try:
subplotLayout = [
None,
(1, 1),
(1, 2),
(2, 2),
(2, 2),
(2, 3),
(2, 3),
(3, 3),
(3, 3),
(3, 3),
][nvar + 1]
except Exception:
raise Exception("No subplot layout for %d plots" % nvar)
if subplotLayout is None:
raise Exception("No output names found")
ax_rmse = subfigs[1].subplots(
nrows=subplotLayout[0],
ncols=subplotLayout[1],
sharex=True,
sharey=True,
squeeze=False,
)
ax_rmse = [item for row in ax_rmse for item in row] # Flatten
ax_rmse[0].set_xlim(-1, epoch + 1)
ax_rmse[0].set_ylim(0, ymaxL)
ax_rmse[0].set_ylabel("Variance fraction", fontsize=comp_font_size)
ax_rmse[nvar].set_xlabel("Epoch", fontsize=comp_font_size)
for varI in range(nvar):
ax_rmse[varI].tick_params(axis="both", labelsize=comp_font_size)
ax_rmse[varI].set_title(hts["OutputNames"][varI], fontsize=comp_font_size)
ax_rmse[varI].grid(color=(0, 0, 0, 1), linestyle="-", linewidth=0.1)
addLine(ax_rmse[varI], hts, "Train_RMSE", (1, 0.5, 0.5, 1), 10, idx=varI)
addLine(ax_rmse[varI], hts, "Test_RMSE", (1, 0, 0, 1), 20, idx=varI)
if specification["trainingMask"] is not None:
addLine(
ax_rmse[varI], hts, "Train_RMSE_masked", (0.5, 0.5, 1, 1), 10, idx=varI
)
addLine(ax_rmse[varI], hts, "Test_RMSE_masked", (0, 0, 1, 1), 20, idx=varI)
if chts is not None:
for idx in range(len(chts["OutputNames"])):
if chts["OutputNames"][idx] == hts["OutputNames"][varI]:
addLine(
ax_rmse[varI], chts, "Train_RMSE", (0.5, 0.5, 1, 1), 10, idx=idx
)
addLine(ax_rmse[varI], chts, "Test_RMSE", (0, 0, 1, 1), 20, idx=idx)
break
ax_rmse[nvar].tick_params(axis="both", labelsize=comp_font_size)
ax_rmse[nvar].set_title("Regularization", fontsize=comp_font_size)
ax_rmse[nvar].grid(color=(0, 0, 0, 1), linestyle="-", linewidth=0.1)
addLine(ax_rmse[nvar], hts, "Regularization_loss", (1, 0, 0, 1), 20)
if chts is not None:
addLine(ax_rmse[nvar], chts, "Regularization_loss", (0, 0, 1, 1), 20)
for varI in range(nvar + 1, len(ax_rmse)):
ax_rmse[varI].set_axis_off()
# Right - KL-divergence plots
ax_kld = subfigs[2].subplots(
nrows=2,
ncols=1,
sharex=True,
sharey=False,
)
ax_kld[0].set_xlim(-1, epoch + 1)
ymaxL = max(hts["Train_logpz"] + hts["Test_logpz"])
yminL = min(hts["Train_logpz"] + hts["Test_logpz"])
if chts is not None:
ymaxL = max(ymaxL, max(chts["Train_logpz"] + chts["Test_logpz"]))
yminL = min(yminL, min(chts["Train_logpz"] + chts["Test_logpz"]))
ymaxL += (ymaxL - yminL) / 20
yminL -= (ymaxL - yminL) / 21
ax_kld[0].set_ylim(yminL, ymaxL)
ax_kld[0].set_title("KL Divergence")
ax_kld[0].set_ylabel("logpz")
ax_kld[0].set_xlabel("")
ax_kld[0].grid(color=(0, 0, 0, 1), linestyle="-", linewidth=0.1)
addLine(ax_kld[0], hts, "Train_logpz", (1, 0.5, 0.5, 1), 10)
addLine(ax_kld[0], hts, "Test_logpz", (1, 0, 0, 1), 20)
if chts is not None:
addLine(ax_kld[0], chts, "Train_logpz", (0.5, 0.5, 1, 1), 10)
addLine(ax_kld[0], chts, "Test_logpz", (0, 0, 1, 1), 20)
ymaxL = max(hts["Train_logqz_x"] + hts["Test_logqz_x"])
yminL = min(hts["Train_logqz_x"] + hts["Test_logqz_x"])
if chts is not None:
ymaxL = max(ymaxL, max(chts["Train_logqz_x"] + chts["Test_logqz_x"]))
yminL = min(yminL, min(chts["Train_logqz_x"] + chts["Test_logqz_x"]))
ymaxL += (ymaxL - yminL) / 20
yminL -= (ymaxL - yminL) / 21
ax_kld[1].set_ylim(yminL, ymaxL)
ax_kld[1].set_ylabel("logqz_x")
ax_kld[1].set_xlabel("epoch")
ax_kld[1].grid(color=(0, 0, 0, 1), linestyle="-", linewidth=0.1)
addLine(ax_kld[1], hts, "Train_logqz_x", (1, 0.5, 0.5, 1), 10)
addLine(ax_kld[1], hts, "Test_logqz_x", (1, 0, 0, 1), 20)
if chts is not None:
addLine(ax_kld[1], chts, "Train_logqz_x", (0.5, 0.5, 1, 1), 10)
addLine(ax_kld[1], chts, "Test_logqz_x", (0, 0, 1, 1), 20)
# Output as png
fig.savefig(fileName)
# Get target and encoded scalar statistics for one test case
def computeScalarStats(
specification, x, generated, min_lat=-90, max_lat=90, min_lon=-180, max_lon=180
):
nFields = specification["nOutputChannels"]
# get the date from the filename tensor
dateStr = x[0][0].numpy().decode("utf-8")
year = int(dateStr[:4])
month = int(dateStr[5:7])
dtp = datetime.date(year, month, 15)
def tensor_to_cube(t):
result = grids.E5sCube.copy()
result.data = np.squeeze(t.numpy())
result.data = np.ma.masked_where(result.data == 0.0, result.data, copy=False)
return result
def field_to_scalar(field):
field = field.extract(
iris.Constraint(
coord_values={"grid_latitude": lambda cell: min_lat <= cell <= max_lat}
)
& iris.Constraint(
coord_values={"grid_longitude": lambda cell: min_lon <= cell <= max_lon}
)
)
return np.mean(field.data)
stats = {}
stats["dtp"] = dtp
stats["target"] = {}
stats["generated"] = {}
for varI in range(nFields):
if specification["trainingMask"] is None:
stats["target"][specification["outputNames"][varI]] = field_to_scalar(
tensor_to_cube(tf.squeeze(x[-1][:, :, :, varI])),
)
stats["generated"][specification["outputNames"][varI]] = field_to_scalar(
tensor_to_cube(tf.squeeze(generated[:, :, :, varI])),
)
else:
mask = specification["trainingMask"].numpy().squeeze()
stats["target"][specification["outputNames"][varI]] = field_to_scalar(
tensor_to_cube(tf.squeeze(x[-1][:, :, :, varI] * mask)),
)
stats["target"]["%s_masked" % specification["outputNames"][varI]] = (
field_to_scalar(
tensor_to_cube(tf.squeeze(x[-1][:, :, :, varI] * (1 - mask))),
)
)
stats["generated"][specification["outputNames"][varI]] = field_to_scalar(
tensor_to_cube(tf.squeeze(generated[:, :, :, varI] * mask)),
)
stats["generated"]["%s_masked" % specification["outputNames"][varI]] = (
field_to_scalar(
tensor_to_cube(tf.squeeze(generated[:, :, :, varI] * (1 - mask))),
)
)
return stats
def plotScalarStats(all_stats, specification, fileName="multi.webp"):
nFields = specification["nOutputChannels"]
if specification["trainingMask"] is not None:
nFields *= 2
figScale = 3.0
wRatios = (3, 1.25)
# Make the plot
fig = Figure(
figsize=(figScale * sum(wRatios), figScale * nFields),
dpi=300,
facecolor=(1, 1, 1, 1),
edgecolor=None,
linewidth=0.0,
frameon=True,
subplotpars=None,
tight_layout=None,
)
canvas = FigureCanvas(fig)
font = {
"family": "DejaVu Sans",
"sans-serif": "DejaVu Sans",
"weight": "normal",
"size": 14,
}
matplotlib.rc("font", **font)
# Plot a variable in its subfigure
def plot_var(sfig, ts, t, m, label):
# Get two axes in the subfig
var_axes = sfig.subplots(nrows=1, ncols=2, width_ratios=wRatios, squeeze=False)
# Calculate y range
ymin = min(min(t), min(m))
ymax = max(max(t), max(m))
ypad = (ymax - ymin) * 0.1
if ypad == 0:
ypad = 1
# First subaxis for time-series plot
var_axes[0, 0].set_xlim(
ts[0] - datetime.timedelta(days=15), ts[-1] + datetime.timedelta(days=15)
)
var_axes[0, 0].set_ylim(ymin - ypad, ymax + ypad)
var_axes[0, 0].grid(color=(0, 0, 0, 1), linestyle="-", linewidth=0.1)
var_axes[0, 0].text(
ts[0] - datetime.timedelta(days=15),
ymax + ypad,
label,
ha="left",
va="top",
bbox=dict(boxstyle="square,pad=0.5", fc=(1, 1, 1, 1)),
zorder=100,
)
var_axes[0, 0].add_line(
Line2D(ts, t, linewidth=2, color=(0, 0, 0, 1), alpha=1.0, zorder=50)
)
var_axes[0, 0].add_line(
Line2D(ts, m, linewidth=2, color=(1, 0, 0, 1), alpha=1.0, zorder=60)
)
# Second subaxis for scatter plot
var_axes[0, 1].set_xlim(ymin - ypad, ymax + ypad),
var_axes[0, 1].set_ylim(ymin - ypad, ymax + ypad),
var_axes[0, 1].grid(color=(0, 0, 0, 1), linestyle="-", linewidth=0.1)
var_axes[0, 1].scatter(t, m, s=2, color=(1, 0, 0, 1), zorder=60)
var_axes[0, 1].add_line(
Line2D(
(ymin - ypad, ymax + ypad),
(ymin - ypad, ymax + ypad),
linewidth=1,
color=(0, 0, 0, 1),
alpha=0.2,
zorder=10,
)
)
# Each variable in its own subfig
subfigs = fig.subfigures(nFields, 1, wspace=0.01)
if nFields == 1:
subfigs = [subfigs]
for varI in range(len(specification["outputNames"])):
if specification["trainingMask"] is None:
vName = specification["outputNames"][varI]
plot_var(
subfigs[varI],
all_stats["dtp"],
all_stats["target"][vName],
all_stats["generated"][vName],
vName,
)
else:
vName = specification["outputNames"][varI]
plot_var(
subfigs[varI * 2],
all_stats["dtp"],
all_stats["target"][vName],
all_stats["generated"][vName],
vName,
)
vName = "%s_masked" % specification["outputNames"][varI]
plot_var(
subfigs[varI * 2 + 1],
all_stats["dtp"],
all_stats["target"][vName],
all_stats["generated"][vName],
specification["outputNames"][varI] + " (masked)",
)
fig.savefig(fileName)